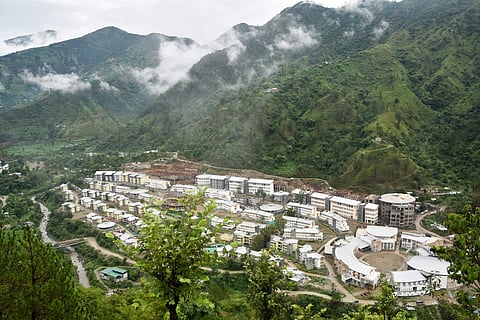
A researcher at the Indian Institute of Technology (IIT) Mandi has proposed sampling techniques to accurately provide insight into the real world high dimensional datasets.
Rameshwar Pratap, Assistant Professor at IIT Mandi in collaboration with Microsoft Research India, Bengaluru, and Carnegie Mellon University, Pittsburgh, USA, has proposed simple, efficient, and accurate sampling techniques to provide insight in the real world high dimensional datasets.
According to Pratap, recent technological advancements in the world have generated a large volume of high dimensional datasets from various sources such as Internet of Things (IoT), World Wide Web, bioinformatics, finance, social network, smart home appliances, smart cities and 5G communication media, among others.
"These high dimensional datasets need to be carefully analysed to infer interesting insights that can be useful for making important decisions," Pratap said.
"Typically several algorithmic techniques such as clustering, regression, and classification are used to analyse Big data. However one of the major challenges in the real-world datasets is that they consist of outliers or anomalies which potentially can confuse these algorithms, and consequently can lead to incorrect insights," he said.
To address these challenges, the researchers have come up with simple techniques for two fundamental unsupervised learning tasks -- Clustering and Dimensionality Reduction.
"As both clustering and principal component analysis are fundamental subroutines in many artificial intelligence applications such as text, audio, video and image compression, building scalable recommendation systems, faster duplicate detection, scalable indexing for faster search, and many more, our results can potentially get accurate and scalable solutions in all of these applications, even when the data is noisy," he said.
In clustering, the research has focused on a famous clustering algorithm 'k-means' clustering.
"In this clustering, the aim is to group the data points into k number of clusters such that points belonging to a particular cluster are more closer to its cluster centre than the remaining. Finding the optimal clustering is hard," he said.
Sharing details of his research, Pratap said in order to address this challenge efficient sampling algorithms have been proposed so that output is close to the optimal solution -- approximate representative of each cluster centre.
"However, the presence of outliers can confuse the sampling algorithm that in turn may output a solution which is very far from the optimal.
To address this, researchers have proposed a sampling algorithm which can efficiently find a close to optimal clustering solution even when outliers are present in the datasets.
"As the presence of outliers can confuse these sampling algorithms and the resulting solution can be significantly worse, we have proposed efficient and accurate sampling algorithms which find close to optimal principal components even when outliers are present in the datasets," he said.